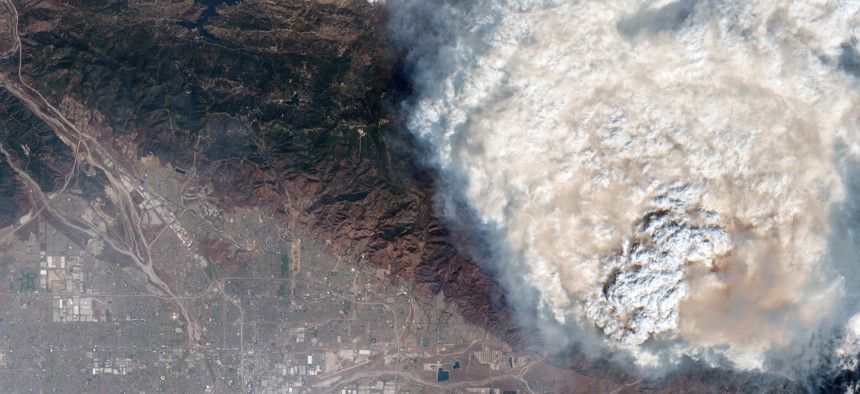
Satellite view of smoke plumes from the ongoing Line Fire in the Angeles National Forest, Calif. NASA, IBM and Oak Ridge National Laboratory teamed up on a foundational AI weather model trained on 40 years of historical weather data. Gallo Images/Getty Images
IBM, NASA and Oak Ridge develop a new AI foundation model for weather
The foundation artificial intelligence model will handle specific meteorological data to offer improved accuracy in weather patterns and forecasting.
A new foundation model for climate- and weather-focused AI capabilities has been developed by IBM in partnership with the National Aeronautics and Space Administration and Oak Ridge National Laboratory, the company announced Monday. The new model is designed to process data specific to climate modeling, in order to detect and predict severe weather patterns, improve the spatial resolution of global climate simulations and improve how physical processes are represented in numerical weather and climate models.
Beyond these applications, the foundation model is also designed to be scalable for other short- and long-term weather projections. The model is available for download on Hugging Face, an open source AI code repository. It is part of the proprietary IBM-NASA Prithvi family of AI foundation models, a weather-specific model series whose name stems from the Sanskrit word for “Earth.”
“Advancing NASA’s Earth science for the benefit of humanity means delivering actionable science in ways that are useful to people, organizations, and communities. The rapid changes we’re witnessing on our home planet demand this strategy to meet the urgency of the moment,” said Karen St. Germain, director of the Earth Science Division of NASA’s Science Mission Directorate, in a press release. “The NASA foundation model will help us produce a tool that people can use: weather, seasonal, and climate projections to help inform decisions on how to prepare, respond, and mitigate.”
Trained on 40 years of Earth observation data from NASA's Modern-Era Retrospective analysis for Research and Applications, researchers say the model has a specialized architecture that keeps it adjustable for a diverse amount of global, regional and local weather modeling applications.
One study, documented in a paper unveiling the foundation model, reportedly showed its ability to accurately reconstruct global surface temperatures using a sample data set derived from just 5% original data.
There are two specific tasks the model is designed and trained to handle. One is climate and weather downscaling, which features inferring high-resolution outputs from low-resolution inputs. Data for these types of problems includes temperature, weather prediction and surface winds, which all have highly variable resolutions. A model handling these data would translate it at up to twelve times the resolution quality, regardless of the resolution of the input data.
The second application, gravity wave parameterization, focuses on using existing numerical climate models and data to illustrate the atmosphere’s gravity waves. Improved gravity wave generation will in turn improve the accuracy of input numerical weather models through better estimations of gravity wave generation and its impact.
“This space has seen the emergence of large AI models that focus on a fixed dataset and single use case — primarily forecasting,” said Juan Bernabe-Moreno, the director of IBM Research Europe and IBM's Accelerated Discovery Lead for Climate and Sustainability. “We have designed our weather and climate foundation model to go beyond such limitations so that it can be tuned to a variety of inputs and uses.”
This collaboration moves in sync with the Biden administration’s efforts to prioritize the innovation stemming from public-private sector partnerships.